- Добавил: literator
- Дата: 16-06-2025, 20:38
- Комментариев: 0
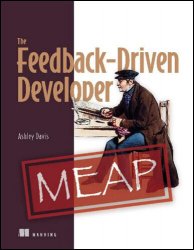
Автор: Ashley Davis
Издательство: Manning Publications
Год: 2025
Страниц: 186
Язык: английский
Формат: pdf, epub
Размер: 19.0 MB
Build habits and processes to deliver quality applications quickly and consistently. The Feedback-Driven Developer teaches you how to build an efficient and effective development process that’s powered by continuous feedback from tests, clients, and your own assessments. Reliable and regular feedback is the core of successful software development. In The Feedback-Driven Developer, you'll learn how to establish the kind of development process that helps you eliminate wasted time and ensure what you're delivering is working and reliable. This entertaining and personal book introduces dozens of practical techniques for using feedback throughout the dev lifecycle book. You’ll see each idea demonstrated hands-on as you explore a full-stack web application. You'll learn how to plan for change, effectively refactor, set up and automate tests, and ship your software to production. As you go, you’ll also explore refactoring into desktop and mobile versions of your applications, expanding the reach of your software. For early- and mid-career developers looking to improve the way they build software. Examples in jаvascript and TypeScript.