- Добавил: literator
- Дата: 16-05-2023, 18:04
- Комментариев: 0
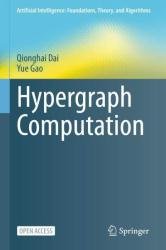
Автор: Qionghai Dai, Yue Gao
Издательство: Springer
Серия: Artificial Intelligence: Foundations, Theory, and Algorithms
Год: 2023
Страниц: 251
Язык: английский
Формат: pdf (true)
Размер: 10.2 MB
Artificial Intelligence (AI) is now everywhere and fuels both industry and daily life all over the world. We are in the era of “Big Data,” and huge sums of information can be obtained which are too cumbersome for people to process themselves. These Big Data are even with much complex correlations behind them in various areas, such as computer vision and social media. For example, the complex correlations among pixels in an image reveal its semantic information, and different types of correlations among social posts infer the users’ emotions. Therefore, developing effective AI methods to exploit such complex data correlations has become an urgent but challenging task. Graph has been widely used to formulate data correlations. A graph is a non-linear data structure which is composed of groups of vertices and edges, representing the pairwise correlations among vertices. Graph learning and graph neural networks have attracted much attention in both research and industrial fields and become very hot topics in these years. It is noted that the world is far more complex than just pairwise connections, and thus graph-based methods still have limitations on high-order correlation modeling. Hypergraph, as a generation of graph, is able to formulate such high-order correlations among the data and has been investigated in last decades. Recent years have witnessed a great popularity of research on hypergraph-related AI methods, which have been used in computer vision, social media analysis, and etc.