Securing the Connected World: Exploring Emerging Threats and Innovative Solutions
- Добавил: literator
- Дата: 14-04-2025, 04:34
- Комментариев: 0
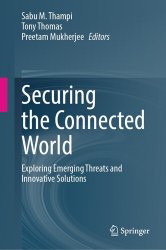
Автор: Sabu M Thampi, Tony Thomas, Preetam Mukherjee
Издательство: Springer
Год: 2025
Страниц: 486
Язык: английский
Формат: pdf (true), epub
Размер: 40.5 MB
Securing the Connected World: Exploring Emerging Threats and Innovative Solutions offers a detailed examination of the growing challenges and cutting-edge solutions in the realms of IoT (Internet of Things) and IoD (Internet of Drones). The book is structured to provide a balanced blend of foundational knowledge and advanced research insights, making it an essential resource for researchers, industry professionals, and students. Covering both established concepts and the latest advancements, it addresses the pressing need for robust security frameworks in today's interconnected digital ecosystems.
The first section of the book lays a strong groundwork for understanding IoT security, exploring areas such as attack modelling, intrusion detection, fraud prevention, and secure communication protocols. It also discusses advanced defenses for 5G-powered IoT networks and the integration of Software-Defined Networking (SDN). The second section focuses on IoD, examining critical topics like authentication, trust management, access control, and ethical considerations in drone-based surveillance. By combining theoretical perspectives with practical applications, this book provides a holistic approach to securing the connected world.
The Internet of Things (IoT) has brought about a new era of interconnection in which devices are implanted with sensors, software, and connections, allowing them to gather and exchange data via the Internet. Due to the efficient features and functionalities of IoT, the network is expanding, and a greater number of devices are linked to the Internet. Many of these devices are designed to be low-power, compact, and easy to deploy, which makes their security vulnerabilities more pronounced. Consequently, the IoT network is susceptible to attacks, and attackers frequently exploit the vulnerability to gain unauthorized access. These attacks can have severe consequences, including the disclosure of private information, the destruction of vital infrastructure, and even physical harm. IoT devices increase the number of attack surfaces that can be used by attackers to compromise the integrity, confidentiality, and accessibility of data as they grow more widespread and interconnected. So it is necessary to identify the vulnerability that exists inside the network to prevent attackers. Organizations can address these weaknesses by recognizing them in advance. Failure to identify this will lead to significant loss. By adopting a proactive and ongoing vulnerability analysis strategy, organizations can strengthen their security posture and lower the likelihood of successful attacks.
Attackers often use the easiest paths to get inside the network. After identifying and prioritizing the vulnerabilities, it is important to model the attacker’s pathways in advance to prevent all the possibilities of an attack. Graphs can effectively illustrate vulnerability relations in a network since they are good at depicting relationships between elements. The use of graphs in attack modeling has prevailed for the last several decades. Several graphical representations, such as attack graphs, attack trees, Attack - defense trees, are in use. Among these attack models, attack graphs are most widely used in representing vulnerability relations. The attack graph can show the attacker’s paths to reach the desired system state. The dynamic and quickly changing nature of IoT networks makes it difficult for traditional attack modeling techniques to keep up. This is where the power of Machine Learning (ML) and Deep Learning (DL) comes into play. With the help of enormous datasets, machine, and Deep Learning technologies thrive at finding hidden patterns, spotting abnormalities, and foreseeing future risks with a level of precision and speed that was previously unattainable. Deep Learning and Machine Learning have shown remarkable promise in transforming the cybersecurity environment. Deep Learning uses complicated neural networks to simulate the operations of the human brain, while Machine Learning is characterized by its capacity to learn from data and make informed judgments. Their algorithms can analyze enormous volumes of data, detect subtle patterns, and adjust to new threats making them ideal for providing security in IoT networks. ML and DL methods can assist with the automatic generation of attack graphs based on system parameters and recognized vulnerabilities.
Скачать Securing the Connected World: Exploring Emerging Threats and Innovative Solutions

[related-news] [/related-news]
Внимание
Уважаемый посетитель, Вы зашли на сайт как незарегистрированный пользователь.
Мы рекомендуем Вам зарегистрироваться либо войти на сайт под своим именем.
Уважаемый посетитель, Вы зашли на сайт как незарегистрированный пользователь.
Мы рекомендуем Вам зарегистрироваться либо войти на сайт под своим именем.