Security Framework for The Internet of Things Applications
- Добавил: literator
- Дата: 13-04-2024, 20:13
- Комментариев: 0
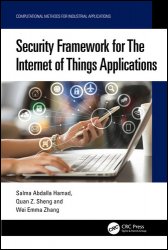
Автор: Salma Abdalla Hamad, Quan Z. Sheng, Wei Emma Zhang
Издательство: CRC Press
Год: 2024
Страниц: 153
Язык: английский
Формат: pdf (true)
Размер: 11.9 MB
The text highlights a comprehensive survey that focuses on all security aspects and challenges facing the Internet of Things systems, including outsourcing techniques for partial computations on edge or cloud while presenting case studies to map security challenges. It further covers three security aspects including Internet of Things device identification and authentication, network traffic intrusion detection, and executable malware files detection.
Industrial Internal of Things (IIoT) products and services collect a large amount of data including those related to users. This collected data are stored either locally on the smart devices or in the cloud. Although Internet of Things (IoT) expansions promise enormous benefits in productivity and efficiency, these devices often lack the security requirements we have become used to in the domain of desktops and server computing. Therefore, processing such large-scale IoT data can also lead to many security issues such as intrusion attacks, data leakage, user privacy, and traceability. The lack of robust security measures in defending IoT systems can compromise the IoT infrastructures and their data. Moreover, since IoT devices usually operate undertight resource constraints, IoT devices are a fruitful target for adversaries’ exploitation.
This book presents substantial contributions to research on building a scalable, real-time IoT security framework. This book provides several novel strategies for identifying and authenticating devices, detecting network-known and zero-day attacks against IoT devices, and detecting malware executables targeting IoT and embedded systems. In this book, we consider three security aspects: IoT device identification and authentication, network traffic intrusion detection, and executable malware file detection.
The first key contribution is constructing a comprehensive survey that focuses on all security aspects and challenges facing IoT systems, including outsourcing techniques for partial computations on edge or cloud while presenting case studies to map security challenges and requirements in real IoT case scenarios.
We use Bidirectional Encoder Representations from Transformers (BERT) embedding, the state-of-the-art Natural Language Processing (NLP) method, to extract contextual information within an executable file’s OpCode sequence. The BERT-generated sentence embedding is fed into a hybrid multi-head CNN-BiLSTM-LocAtt Deep Learning (DL) model. The hybrid CNN-BiLSTM-LocAtt model combines the advantages of the Convolutional Neural Network (CNN) and Bidirectional Long Short-Term Memory (BiLSTM) with the benefits of the Local Attention mechanism (LocAtt) to detect malware. DeepWare extracts the semantic and contextual features and captures long-term dependencies between OpCode sequences, improving the detection performance.
This book:
Presents a security framework model design named Behavioral Network Traffic Identification and Novelty Anomaly Detection for the IoT Infrastructures.
Highlights recent advancements in Machine Learning, Deep Learning, and networking standards to boost Internet of Things security.
Builds a near real-time solution for identifying Internet of Things devices connecting to a network using their network traffic traces and providing them with sufficient access privileges.
Develops a robust framework for detecting IoT anomalous network traffic.
Covers an anti-malware solution for detecting malware targeting embedded devices.
It will serve as an ideal text for senior undergraduate and graduate students, and professionals in the fields of electrical engineering, electronics and communication engineering, computer engineering, and information technology.
Скачать Security Framework for The Internet of Things Applications

[related-news] [/related-news]
Внимание
Уважаемый посетитель, Вы зашли на сайт как незарегистрированный пользователь.
Мы рекомендуем Вам зарегистрироваться либо войти на сайт под своим именем.
Уважаемый посетитель, Вы зашли на сайт как незарегистрированный пользователь.
Мы рекомендуем Вам зарегистрироваться либо войти на сайт под своим именем.