Machine Learning for Future Wireless Communications
- Добавил: literator
- Дата: 19-12-2019, 20:09
- Комментариев: 0
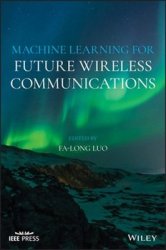
Автор: Fa‐Long Luo
Издательство: Wiley-IEEE Press
Год: 2020
Страниц: 475
Язык: английский
Формат: pdf (true)
Размер: 20.9 MB
A comprehensive review to the theory, application and research of Machine Learning for future wireless communications.
In one single volume, Machine Learning for Future Wireless Communications provides a comprehensive and highly accessible treatment to the theory, applications and current research developments to the technology aspects related to Machine Learning (ML) for wireless communications and networks. The technology development of Machine Learning for wireless communications has grown explosively and is one of the biggest trends in related academic, research and industry communities.
Deep neural networks-based Machine Learning technology is a promising tool to attack the big challenge in wireless communications and networks imposed by the increasing demands in terms of capacity, coverage, latency, efficiency flexibility, compatibility, quality of experience and silicon convergence. The author – a noted expert on the topic – covers a wide range of topics including system architecture and optimization, physical-layer and cross-layer processing, air interface and protocol design, beamforming and antenna configuration, network coding and slicing, cell acquisition and handover, scheduling and rate adaption, radio access control, smart proactive caching and adaptive resource allocations. Uniquely organized into three categories: Spectrum Intelligence, Transmission Intelligence and Network Intelligence, this important resource:
Offers a comprehensive review of the theory, applications and current developments of machine learning for wireless communications and networks
Covers a range of topics from architecture and optimization to adaptive resource allocations
Reviews state-of-the-art machine learning based solutions for network coverage
Includes an overview of the applications of machine learning algorithms in future wireless networks
Explores flexible backhaul and front-haul, cross-layer optimization and coding, full-duplex radio, digital front-end (DFE) and radio-frequency (RF) processing
Written for professional engineers, researchers, scientists, manufacturers, network operators, software developers and graduate students, Machine Learning for Future Wireless Communications presents in 21 chapters a comprehensive review of the topic authored by an expert in the field.
Due to its powerful nonlinear mapping and distribution processing capability, deep NN-based machine learning technology is being considered as a very promising tool to attack the big challenge in wireless communications and networks imposed by the explosively increasing demands in terms of capacity, coverage, latency, efficiency (power, frequency spectrum, and other resources), flexibility, compatibility, quality of experience, and silicon convergence.
Mainly categorized into supervised learning, unsupervised learning, and reinforcement learning, various machine learning (ML) algorithms can be used to provide better channel modeling and estimation in millimeter and terahertz bands; to select a more adaptive modulation (waveform, coding rate, bandwidth, and filtering structure) in massive multiple-input and multiple-output (MIMO) technology; to design more efficient front-end and radio-frequency processing (pre-distortion for power amplifier compensation, beamforming configuration, and crest-factor reduction); to deliver a better compromise in self-interference cancellation for full-duplex transmissions and device-to-device communications; and to offer a more practical solution for intelligent network optimization, mobile edge computing, networking slicing, and radio resource management related to wireless big data, mission-critical communications, massive machine-type communications, and tactile Internet.
Contents:
Скачать Machine Learning for Future Wireless Communications

[related-news] [/related-news]
Внимание
Уважаемый посетитель, Вы зашли на сайт как незарегистрированный пользователь.
Мы рекомендуем Вам зарегистрироваться либо войти на сайт под своим именем.
Уважаемый посетитель, Вы зашли на сайт как незарегистрированный пользователь.
Мы рекомендуем Вам зарегистрироваться либо войти на сайт под своим именем.