- Добавил: literator
- Дата: 12-03-2025, 06:51
- Комментариев: 0
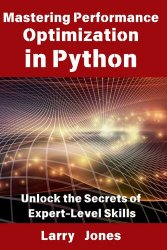
Автор: Larry Jones
Издательство: Walzone Press
Год: 2025
Страниц: 500
Язык: английский
Формат: epub (true)
Размер: 10.1 MB
Dive deep into the art and science of Python performance enhancement with "Mastering Performance Optimization in Python: Unlock the Secrets of Expert-Level Skills." This advanced guide transforms seasoned developers into Python performance experts by exploring sophisticated techniques that elevate coding prowess. From runtime environments and data handling to memory management and concurrency, this book provides the essential insights needed to navigate and conquer complex performance challenges. Each chapter meticulously breaks down intricate concepts, presenting clear, actionable strategies for real-world application. Discover how to leverage Python's built-in libraries, utilize asynchronous programming, and implement multithreading and multiprocessing for optimal efficiency. With a focus on profiling, benchmarking, and continuous monitoring, the book ensures that readers develop the skills to not only identify but also rectify performance bottlenecks, delivering applications that are both robust and scalable.