Causal AI (Final Release)
- Добавил: literator
- Дата: 13-05-2025, 20:22
- Комментариев: 0
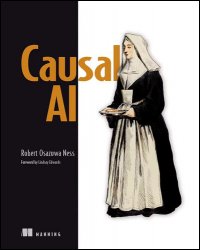
Автор: Robert Osazuwa Ness
Издательство: Manning Publications
Год: 2025
Страниц: 520
Язык: английский
Формат: epub (true)
Размер: 19.2 MB
Build AI models that can reliably deliver causal inference.
How do you know what might have happened, had you done things differently? Causal AI gives you the insight you need to make predictions and control outcomes based on causal relationships instead of pure correlation, so you can make precise and timely interventions. Causal AI is a practical introduction to building AI models that can reason about causality.
In Causal AI you will learn how to:
Build causal reinforcement learning algorithms
Implement causal inference with modern probabilistic machine tools such as PyTorch and Pyro
Compare and contrast statistical and econometric methods for causal inference
Set up algorithms for attribution, credit assignment, and explanation
Convert domain expertise into explainable causal models
Author Robert Osazuwa Ness, a leading researcher in causal AI at Microsoft Research, brings his unique expertise to this cutting-edge guide. His clear, code-first approach explains essential details of causal machine learning that are hidden in academic papers. Everything you learn can be easily and effectively applied to industry challenges, from building explainable causal models to predicting counterfactual outcomes.
about the technology
Traditional ML models can’t answer causal questions like, “Why did that happen?” or, “What factors should I change to get a particular outcome?” This book blends advanced statistical methods, computational techniques, and new algorithms to create machine learning systems that automate the process of causal inference.
about the book
Causal AI introduces the tools, techniques, and algorithms of causal reasoning for Machine Learning. This unique book masterfully blends Bayesian and probabilistic approaches to causal inference with practical hands-on examples in Python. Along the way, you’ll learn to integrate causal assumptions into Deep Learning architectures, including reinforcement learning and large language models. You’ll also use PyTorch, Pyro, and other ML libraries to scale up causal inference.
This book assumes you are familiar with Data Science scripting in Python. The three open source Python libraries we rely on in this book are DoWhy, pgmpy, and Pyro. DoWhy is a library for the open source PyWhy suite of Python libraries for causal inference. pgmpy is a probabilistic graphical modeling library built on SciPy and NetworkX. Pyro is a probabilistic machine learning library that extends PyTorch.
Our code-first goal is unique because, rather than going deep into the statistical theory needed to do causal inference, we rely on these supporting libraries to do the statistics for us. DoWhy tries to be as end-to-end as possible in terms of mapping domain knowledge inputs to causal inference outputs. When we want to do more bespoke modeling, we’ll use pgmpy or Pyro. These libraries provide probabilistic inference algorithms that take care of the estimation theory. pgmpy has graph-based inference algorithms that are extremely reliable. Pyro, as an extension of PyTorch, extends causal modeling to deep generative models on high dimensional data and variational inference—a cutting-edge deep learning–based inference technique.
If your background is in R or Julia, you should still find this book useful. There are numerous R and Julia packages that overlap in functionality with DoWhy. Graphical modeling software in these languages, such as bnlearn, can substitute for pgmpy. Similarly, the ideas we develop with Pyro will work with similar probabilistic programming languages, such as PyMC.
what's inside
End-to-end causal inference with DoWhy
Deep Bayesian causal generative AI models
A code-first tour of the do-calculus and Pearl’s causal hierarchy
Code for fine-tuning causal large language models
about the reader
For data scientists and Machine Learning engineers. Examples in Python.
Скачать Causal AI (Final Release)

[related-news] [/related-news]
Внимание
Уважаемый посетитель, Вы зашли на сайт как незарегистрированный пользователь.
Мы рекомендуем Вам зарегистрироваться либо войти на сайт под своим именем.
Уважаемый посетитель, Вы зашли на сайт как незарегистрированный пользователь.
Мы рекомендуем Вам зарегистрироваться либо войти на сайт под своим именем.