Mathematical Optimization for Machine Learning: Proceedings of the Math+ Thematic Einstein Semester 2023
- Добавил: literator
- Дата: 10-05-2025, 20:46
- Комментариев: 0
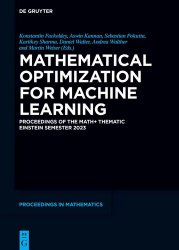
Автор: Konstantin Fackeldey, Aswin Kannan, Sebastian Pokutta, Kartikey Sharma, Daniel Walter
Издательство: De Gruyter
Год: 2025
Страниц: 212
Язык: английский
Формат: pdf (true), epub
Размер: 27.5 MB
Mathematical optimization and Machine Learning are closely related. This proceedings volume of the Thematic Einstein Semester 2023 of the Berlin Mathematics Research Center MATH+ collects recent progress on their interplay in topics such as discrete optimization, nonlinear programming, optimal control, first-order methods, multilevel optimization, Machine Learning in optimization, physics-informed learning, and fairness in Machine Learning.
Mathematical optimization often focuses on accuracy, computational efficiency, and robustness while Machine Learning (ML) aims to achieve effective results on real data sets, in particular concentrating on generalization, robustness, and resilience (to, e.g., perturbations of the inputs). Up to now, both research areas have been only loosely intertwined with optimization being a tool to execute a learning task from the ML perspective, and ML being just yet another application from the optimization perspective. This is illustrated by the fact that certain variants of stochastic gradient descent (e. g., out-of-the-box Adam) are still the method of choice in ML even though the application of this method is not justified by theory for a large number of methods from ML. On the other hand, mathematical optimization often studies approximation properties for Machine Learning tasks but so far has not developed optimization approaches that deliberately target the needs of ML (e.g., generalization or sparsity). Hence, there is a lack of advanced optimization techniques that can handle Machine Learning problems with the required efficiency or learning-based improvements to optimization. Connecting mathematical optimization perspectives with Machine Learning approaches can lead to new approaches and benefit existing ones. For instance, the problem of predicting cluster membership in unsupervised learning may be greatly aided by an optimization perspective; after all, as with many other Data Science approaches, the problem can be viewed as maximizing a (complicated) function subject to some constraints. Complementarily, ML can be used to tune optimization methods or develop surrogate models. It heavily influences downstream decision-making and more general decision support systems in many real-world applications across industries.
Скачать Mathematical Optimization for Machine Learning: Proceedings of the Math+ Thematic Einstein Semester 2023

[related-news] [/related-news]
Внимание
Уважаемый посетитель, Вы зашли на сайт как незарегистрированный пользователь.
Мы рекомендуем Вам зарегистрироваться либо войти на сайт под своим именем.
Уважаемый посетитель, Вы зашли на сайт как незарегистрированный пользователь.
Мы рекомендуем Вам зарегистрироваться либо войти на сайт под своим именем.