Advances in Optimization Algorithms for Multidisciplinary Engineering Applications
- Добавил: literator
- Дата: 26-04-2025, 18:43
- Комментариев: 0
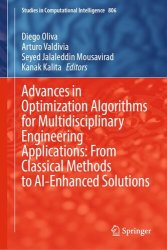
Автор: Diego Oliva, Arturo Valdivia, Seyed Jalaleddin Mousavirad, Kanak Kalita
Издательство: Springer
Серия: Studies in Computational Intelligence
Год: 2025
Страниц: 798
Язык: английский
Формат: pdf (true)
Размер: 48.9 MB
This book is an authoritative compilation of the latest advancements in optimization techniques. This book covers a wide array of methods ranging from classical to metaheuristic to AI-enhanced approaches.
The chapters are meticulously selected and organized in three sections—metaheuristics, Machine Learning and engineering applications. This allows for an in-depth exploration of diverse topics ranging from image processing to feature selection to data clustering, to practical applications like energy optimization, smart grids, healthcare diagnostics, etc. Each chapter delves into the specific algorithms and applications as well as provides ample theoretical insights.
Accordingly, this book is ideally suited for undergraduate and postgraduate students in fields such as science, engineering and computational mathematics. It is also an invaluable resource for courses on Artificial Intelligence, Computational Intelligence, etc. Researchers and professionals in evolutionary computation, Artificial Intelligence and engineering will find the material especially useful for advancing their work and exploring new frontiers in optimization.
Metaheuristic algorithms have gained popularity in addressing various optimization challenges in diverse fields such as digital image processing, energy, machine learning, robotics, and data analytics. Most metaheuristic methods are population-based, where a population of search agents (or individuals) explores different candidate solutions within a solution space. These optimization frameworks offer several advantages, including the interaction between individuals, which is crucial for effectively exploring the search space and overcoming local optima. Metaheuristic algorithms must balance exploring the search space for promising new solutions and exploiting regions containing high-quality solutions. Clustering and partitioning help divide the search space into smaller, more manageable regions, allowing effective exploration and intensive exploitation of promising regions. These techniques can be employed in a variety of metaheuristic algorithms. This chapter provides a comprehensive review of clustering and partitioning techniques within metaheuristic algorithms. Its purpose is to provide a detailed analysis of these techniques, identify their applications, advantages, and limitations, and facilitate their understanding and use in optimization problems.
Contents:
Скачать Advances in Optimization Algorithms for Multidisciplinary Engineering Applications

[related-news] [/related-news]
Внимание
Уважаемый посетитель, Вы зашли на сайт как незарегистрированный пользователь.
Мы рекомендуем Вам зарегистрироваться либо войти на сайт под своим именем.
Уважаемый посетитель, Вы зашли на сайт как незарегистрированный пользователь.
Мы рекомендуем Вам зарегистрироваться либо войти на сайт под своим именем.