Graph Neural Networks in Action (Final Release)
- Добавил: literator
- Дата: 19-02-2025, 17:52
- Комментариев: 0
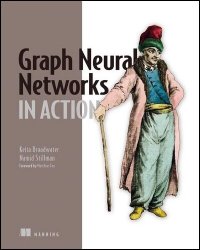
Автор: Keita Broadwater, Namid Stillman
Издательство: Manning Publications
Год: 2025
Страниц: 394
Язык: английский
Формат: pdf (true)
Размер: 18.3 MB
A hands-on guide to powerful graph-based Deep Learning models.
In Graph Neural Networks in Action, you will learn how to:
Train and deploy a graph neural network
Generate node embeddings
Use GNNs at scale for very large datasets
Build a graph data pipeline
Create a graph data schema
Understand the taxonomy of GNNs
Manipulate graph data with NetworkX
Graph Neural Networks in Action teaches you to create powerful Deep Learning models for working with graph data. You’ll learn how to both design and train your models, and how to develop them into practical applications you can deploy to production. Go hands-on and explore relevant real-world projects as you dive into graph neural networks perfect for node prediction, link prediction, and graph classification. Inside this practical guide, you’ll explore common graph neural network architectures and cutting-edge libraries, all clearly illustrated with well-annotated Python code.
About the technology:
Graph neural networks expand the capabilities of Deep Learning beyond traditional tabular data, text, and images. This exciting new approach brings the amazing capabilities of Deep Learning to graph data structures, opening up new possibilities for everything from recommendation engines to pharmaceutical research.
About the book:
In Graph Neural Networks in Action you’ll create deep learning models that are perfect for working with interconnected graph data. Start with a comprehensive introduction to graph data’s unique properties. Then, dive straight into building real-world models, including GNNs that can generate node embeddings from a social network, recommend eCommerce products, and draw insights from social sites. This comprehensive guide contains coverage of the essential GNN libraries, including PyTorch Geometric, DeepGraph Library, and Alibaba’s GraphScope for training at scale.
Python is the coding language of choice throughout this book. There are now several GNN libraries in the Python ecosystem, including PyTorch Geometric (PyG), Deep Graph Library (DGL), GraphScope, and Jraph. We focus on PyG, which is one of the most popular and easy-to-use frameworks, written on top of PyTorch. We want this book to be approachable by an audience with a wide set of hardware constraints, so with the exception of some individual sections and chapter 7 on scalability, distributed systems and GPU systems aren’t required, although they can be used for some of the coded examples.
The book provides a survey of the most relevant implementations of GNNs, including graph convolutional networks (GCNs), graph autoencoders (GAEs), graph attention networks (GATs), and graph long short-term memory (LSTM). The aim is to cover the GNN tasks mentioned earlier. In addition, we’ll touch on different types of graphs, including knowledge graphs. This book contains many examples of source code both in numbered listings and in line with normal text.
About the reader:
For Python programmers familiar with Machine Learning and the basics of Deep Learning. If you have experience in object-oriented programming, you’ll find the concepts particularly accessible and applicable.
Скачать Graph Neural Networks in Action (Final Release)

[related-news] [/related-news]
Внимание
Уважаемый посетитель, Вы зашли на сайт как незарегистрированный пользователь.
Мы рекомендуем Вам зарегистрироваться либо войти на сайт под своим именем.
Уважаемый посетитель, Вы зашли на сайт как незарегистрированный пользователь.
Мы рекомендуем Вам зарегистрироваться либо войти на сайт под своим именем.