Supervised Learning: Mathematical Foundations and Real-world Applications
- Добавил: literator
- Дата: Вчера, 19:20
- Комментариев: 0
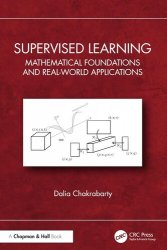
Автор: Dalia Chakrabarty
Издательство: CRC Press
Год: 2025
Страниц: 345
Язык: английский
Формат: pdf (true), epub
Размер: 22.5 MB
This book discusses the relevance of probabilistic Supervised Learning, to the pursuit of automated and reliable prediction of an unknown that is in a state of relationship with another variable. The book provides methods for secured mechanistic learning of the function that represents this relationship between the output and input variables, where said learning is undertaken within the remit of real-world information that can be messy in different ways. For example, the available data may be highly multivariate or be high-dimensional, reflecting the nature of the output variable that could be a vector, or matrix, or even higher in dimension, as is often the case in a real-world application. Additionally, the data is noisy, and often it is small to moderately large in size in multiple applications. Another difficulty that regularly arises is that the training dataset – comprising pairs of values of the input and output –is such, that the sought function cannot be captured by a parametric shape, but is instead underlined by an inhomogeneous correlation structure. These difficulties notwithstanding, we desire a streamlined methodology that allows the learning of the inter variable relationship – to ultimately permit fast and reliable predictions of the output, at newly recorded values of the input. In fact, occasions arise when one seeks values of the input at which a new output value is recorded, and such a demand is also addressed in the book.
The generic solution to the problem of secured supervised learning amidst real-world messiness, lies in treating the sought inter-variable relation as a (function-valued) random variable, which, being random, is ascribed a probability distribution. Then recalling that distributions on the space of functions are given by stochastic processes, the sought function is proposed to be a sample function of a stochastic process. This process is chosen as one that imposes minimal constraints on the sought function – identified as a Gaussian Process (GP) in the book. Thus, the sought function can be inferred upon, as long as the co-variance function of the underlying GP is learnt, given the available training set. The book presents probabilistic techniques to undertake said learning, within the challenges borne by the data, and illustrates such techniques on real data. Learning of a function is always followed by closed-form prediction of the mean and dispersion of the output variable that is realised at a test input.
To help with the background, the book includes reviews on stochastic processes and basic probability theory. This will render the first half of the book useful for students across disciplines, while the latter half will be appreciated by students of numerate subjects at the postgraduate level or higher, including students of computational sciences, statistics and mathematics.
Скачать Supervised Learning: Mathematical Foundations and Real-world Applications

[related-news] [/related-news]
Внимание
Уважаемый посетитель, Вы зашли на сайт как незарегистрированный пользователь.
Мы рекомендуем Вам зарегистрироваться либо войти на сайт под своим именем.
Уважаемый посетитель, Вы зашли на сайт как незарегистрированный пользователь.
Мы рекомендуем Вам зарегистрироваться либо войти на сайт под своим именем.