Statistics for Data Science and Analytics
- Добавил: literator
- Дата: Сегодня, 02:31
- Комментариев: 0
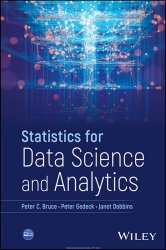
Автор: Peter C. Bruce, Peter Gedeck, Janet Dobbins
Издательство: Wiley
Год: 2025
Страниц: 381
Язык: английский
Формат: True/Retail PDF, True/Retail EPUB
Размер: 29.8 MB
Introductory statistics textbook with a focus on Data Science topics such as prediction, correlation, and data exploration.
Statistics for Data Science and Analytics is a comprehensive guide to statistical analysis using Python, presenting important topics useful for data science such as prediction, correlation, and data exploration. The authors provide an introduction to statistical science and big data, as well as an overview of Python data structures and operations.
A range of statistical techniques are presented with their implementation in Python, including hypothesis testing, probability, exploratory data analysis, categorical variables, surveys and sampling, A/B testing, and correlation. The text introduces binary classification, a foundational element of Machine Learning, validation of statistical models by applying them to holdout data, and probability and inference via the easy-to-understand method of resampling and the bootstrap instead of using a myriad of “kitchen sink” formulas. Regression is taught both as a tool for explanation and for prediction.
Python is a general programming language that can be used in many different areas. It is especially popular in the Machine Learning and Data Science communities. A wide range of libraries provide efficient solutions for almost every need, from simple one-off scripts, to web servers, and highly complex scientific applications. As we will see throughout this book, it also has great support for statistics. You can use Python in many different ways. For most people new to the language, the easiest way to get started is to use Python in Jupyter notebooks. Jupyter notebooks are documents that contain both code and rich text elements, such as figures, links, equations, etc. Because of this, they are an ideal environment to learn Python and to present your work. You will find notebooks with the example code of this book on our website. A great way to get started with Python is to run code on one of the freely accessible cloud computing platforms. Google Colab has a free tier that is sufficient for all the examples in this book.
This book is informed by the authors' experience designing and teaching both introductory statistics and Machine Learning at Statistics.com. Each chapter includes practical examples, explanations of the underlying concepts, and Python code snippets to help readers apply the techniques themselves.
Statistics for Data Science and Analytics includes information on sample topics such as:
Int, float, and string data types, numerical operations, manipulating strings, converting data types, and advanced data structures like lists, dictionaries, and sets
Experiment design via randomizing, blinding, and before-after pairing, as well as proportions and percents when handling binary data
Specialized Python packages like numpy, scipy, pandas, scikit-learn and statsmodels―the workhorses of data science―and how to get the most value from them
Statistical versus practical significance, random number generators, functions for code reuse, and binomial and normal probability distributions
Written by and for Data Science instructors, Statistics for Data Science and Analytics is an excellent learning resource for Data Science instructors prescribing a required intro stats course for their programs, as well as other students and professionals seeking to transition to the Data Science field.
Скачать Statistics for Data Science and Analytics

[related-news] [/related-news]
Внимание
Уважаемый посетитель, Вы зашли на сайт как незарегистрированный пользователь.
Мы рекомендуем Вам зарегистрироваться либо войти на сайт под своим именем.
Уважаемый посетитель, Вы зашли на сайт как незарегистрированный пользователь.
Мы рекомендуем Вам зарегистрироваться либо войти на сайт под своим именем.