Machine Learning under Resource Constraints, Vol. 1-3
- Добавил: literator
- Дата: 14-02-2023, 06:44
- Комментариев: 0
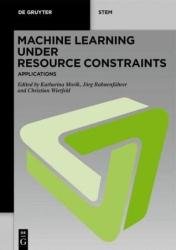
Автор: Katharina Morik, Jorg Rahnenfuhrer, Christian Wietfeld
Издательство: De Gruyter
Год: 2023
Страниц: 506+364+478
Язык: английский
Формат: pdf (true)
Размер: 129.6 MB
"Машинное обучение в условиях ограниченности ресурсов: в трех томах" рассматривает новые алгоритмы машинного обучения, которые сталкиваются с проблемами высокой пропускной способности данных, высокой размерности или сложной структуры данных. Ограничения на ресурсы определяются соотношением между требованиями к обработке данных и возможностями вычислительной техники. Ресурсами являются время выполнения, память, связь и энергия. Следовательно, современные компьютерные архитектуры играют важную роль. Новые алгоритмы машинного обучения оптимизируются с учетом минимального потребления ресурсов. Более того, полученные прогнозы выполняются на различных архитектурах для экономии ресурсов. В книге представлен всеобъемлющий обзор новых подходов к исследованию машинного обучения, учитывающих ограничения ресурсов, а также применение описанных методов в различных областях науки и техники.
Machine Learning under Resource Constraints addresses novel Machine Learning algorithms that are challenged by high-throughput data, by high dimensions, or by complex structures of the data in three volumes. Resource constraints are given by the relation between the demands for processing the data and the capacity of the computing machinery. The resources are runtime, memory, communication, and energy. Hence, modern computer architectures play a significant role. Novel Machine Learning algorithms are optimized with regard to minimal resource consumption. Moreover, learned predictions are executed on diverse architectures to save resources. It provides a comprehensive overview of the novel approaches to Machine Learning research that consider resource constraints, as well as the application of the described methods in various domains of science and engineering.
Volume 1 establishes the foundations of this new field. It goes through all the steps from data collection, their summary and clustering, to the different aspects of resource-aware learning, i.e., hardware, memory, energy, and communication awareness. Several Machine Learning methods are inspected with respect to their resource requirements and how to enhance their scalability on diverse computing architectures ranging from embedded systems to large computing clusters.
Volume 2 covers Machine Learning for knowledge discovery in particle and astroparticle physics. Their instruments, e.g., particle detectors or telescopes, gather petabytes of data. Here, Machine Learning is necessary not only to process the vast amounts of data and to detect the relevant examples efficiently, but also as part of the knowledge discovery process itself. The physical knowledge is encoded in simulations that are used to train the machine learning models. At the same time, the interpretation of the learned models serves to expand the physical knowledge. This results in a cycle of theory enhancement supported by Machine Learning.
Volume 3 describes how the resource-aware Machine Learning methods and techniques are used to successfully solve real-world problems. The book provides numerous specific application examples. In the areas of health and medicine, it is demonstrated how Machine Learning can improve risk modelling, diagnosis, and treatment selection for diseases. Machine Learning supported quality control during the manufacturing process in a factory allows to reduce material and energy cost and save testing times is shown by the diverse real-time applications in electronics and steel production as well as milling. Additional application examples show, how machine-learning can make traffic, logistics and smart cities more effi cient and sustainable. Finally, mobile communications can benefi t substantially from Machine Learning, for example by uncovering hidden characteristics of the wireless channel.
Скачать Machine Learning under Resource Constraints, Volume 1-3

[related-news] [/related-news]
Внимание
Уважаемый посетитель, Вы зашли на сайт как незарегистрированный пользователь.
Мы рекомендуем Вам зарегистрироваться либо войти на сайт под своим именем.
Уважаемый посетитель, Вы зашли на сайт как незарегистрированный пользователь.
Мы рекомендуем Вам зарегистрироваться либо войти на сайт под своим именем.