Latent Factor Analysis for High-dimensional and Sparse Matrices: A particle swarm optimization-based approach
- Добавил: literator
- Дата: 16-11-2022, 12:56
- Комментариев: 0
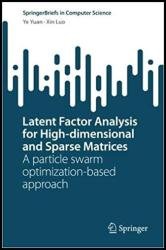
Автор: Ye Yuan, Xin Luo
Издательство: Springer
Год: 2022
Страниц: 99
Язык: английский
Формат: pdf (true), epub
Размер: 22.7 MB
Latent factor analysis models are an effective type of machine learning model for addressing high-dimensional and sparse matrices, which are encountered in many big-data-related industrial applications. The performance of a latent factor analysis model relies heavily on appropriate hyper-parameters. However, most hyper-parameters are data-dependent, and using grid-search to tune these hyper-parameters is truly laborious and expensive in computational terms. Hence, how to achieve efficient hyper-parameter adaptation for latent factor analysis models has become a significant question.
High-dimensional and sparse (HiDS) matrices are commonly seen in many big data-related industrial applications like electronic commerce, cloud services, social networks, and wireless sensor networks. Despite its extreme sparse, an HiDS matrix contains rich knowledge regarding desired patterns like users’ potential favorites, item clusters, and topological neighbors. Hence, how to efficiently and accurately extract desired knowledge from it for various data analysis tasks becomes a highly interesting issue.
Latent factor analysis (LFA) has proven to be highly efficient for addressing an HiDS matrix owing to its high scalability and efficiency. However, an LFA model’s performance relies heavily on its hyper-parameters, which should be chosen with care. The common strategy is to employ grid-search to tune these hyper-parameters. However, it requires expensive computational cost to search the whole candidate space, especially when building an LFA model on an HiDS matrix. Hence, how to implement a hyper-parameter-free LFA model becomes a significant issue.
In this book, we incorporate the principle of particle swarm optimization (PSO) into latent factor analysis, thereby achieving four effective hyper-parameter-free latent factor analysis models. The first model is a Learning rate-free LFA (L2FA) model, which utilizes a standard PSO algorithm into the learning process by building a swarm of learning rates applied to the same group. The second model is a Learning rate and Regularization coefficient-free LFA (LRLFA) model, which build a swarm by taking the learning rate and regularization coefficient of every single LFA-based model as particles, and then apply particle swarm optimization to make them adaptation according to a predefined fitness function. The third model is a Generalized and Adaptive LFA (GALFA) model, which implement self-adaptation of the regularization coefficient and momentum coefficient for excellent practicability via PSO. The last model is an Advanced Learning rate-free LFA (AL2FA) model. Before building this model, we first propose a novel position-transitional particle swarm optimization (P2SO) algorithm by incorporate more dynamic information into the particle’s evolution for preventing premature convergence. And then, a P2SO algorithm is utilized into the training process to make the learning rate adaptation without accuracy loss. Since hyper-parameter adaptation is done within only one full training process, thereby greatly reducing computational cost. Hence, it fits the need of real applications with high scalability and efficiency.
Скачать Latent Factor Analysis for High-dimensional and Sparse Matrices: A particle swarm optimization-based approach

[related-news] [/related-news]
Внимание
Уважаемый посетитель, Вы зашли на сайт как незарегистрированный пользователь.
Мы рекомендуем Вам зарегистрироваться либо войти на сайт под своим именем.
Уважаемый посетитель, Вы зашли на сайт как незарегистрированный пользователь.
Мы рекомендуем Вам зарегистрироваться либо войти на сайт под своим именем.