- Добавил: literator
- Дата: 26-04-2023, 18:34
- Комментариев: 0
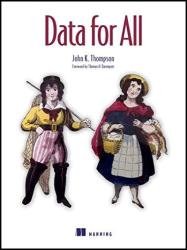
Автор: John K. Thompson
Издательство: Manning Publications
Год: 2023
Страниц: 192
Язык: английский
Формат: pdf (true)
Размер: 10.1 MB
Do you know what happens to your personal data when you are browsing, buying, or using apps? Discover how your data is harvested and exploited, and what you can do to access, delete, and monetize it. Data for All empowers everyone—from tech experts to the general public—to control how third parties use personal data. The data you generate every day is the lifeblood of many large companies—and they make billions of dollars using it. In Data for All, bestselling author John K. Thompson outlines how this one-sided data economy is about to undergo a dramatic change. Thompson pulls back the curtain to reveal the true nature of data ownership, and how you can turn your data from a revenue stream for companies into a financial asset for your benefit. Descriptive statistics are the statistical methods that we learned in high school and college. These are statistical techniques like averages—means, modes, and Gaussian distributions. Data scientists use these tools to create an exploratory data analysis (EDA).