- Добавил: alexcat
- Дата: 15-07-2018, 13:02
- Комментариев: 0
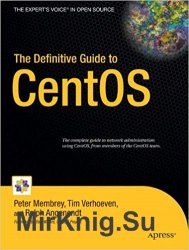
Название: The Definitive Guide to CentOS
Автор: Peter Membrey, Tim Verhoeven, Ralph Angenendt
Издательство: Apress
Год: 2009
Формат: PDF
Страниц: 353
Размер: 10,9 МБ
Язык: English
CentOS is just like Red Hat, but without the price tag and with the virtuous license. When belts have to be tightened, we want to read about an OS with all the features of a commercial Linux variety, but without the pain. The Definitive Guide to CentOS is the first definitive reference for CentOS and focuses on CentOS alone, the workhorse Linux distribution, that does the heavy lifting in small and medium-size enterprises without drawing too much attention to itself.