Data Science for Genomics
- Добавил: literator
- Дата: 21-04-2023, 05:52
- Комментариев: 0
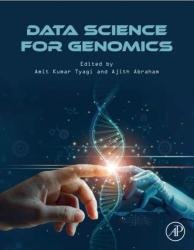
Автор: Amit Kumar Tyagi, Ajith Abraham
Издательство: Academic Press/Elsevier
Год: 2023
Страниц: 314
Язык: английский
Формат: pdf (true)
Размер: 13.6 MB
Data Science for Genomics presents the foundational concepts of data science as they pertain to genomics, encompassing the process of inspecting, cleaning, transforming, and modeling data with the goal of discovering useful information, suggesting conclusions and supporting decision-making. Sections cover Data Science, Machine Learning, Deep Learning, data analysis, and visualization techniques. The authors then present the fundamentals of Genomics, Genetics, Transcriptomes and Proteomes as basic concepts of molecular biology, along with DNA and key features of the human genome, as well as the genomes of eukaryotes and prokaryotes.
Techniques that are more specifically used for studying genomes are then described in the order in which they are used in a genome project, including methods for constructing genetic and physical maps. DNA sequencing methodology and the strategies used to assemble a contiguous genome sequence and methods for identifying genes in a genome sequence and determining the functions of those genes in the cell. Readers will learn how the information contained in the genome is released and made available to the cell, as well as methods centered on cloning and PCR.
Automated ML, abbreviated and popularly known as AutoML, is a process of applying automation to the ML life cycle with the aim to automate the repetitive tasks of it. This will provide an edge to the technology by not only democratizing it and making it accessible to all but will have various other advantages like reduction of the model run time, a well-tuned model, various evaluation metrics to judge the model performance, etc. Traditional ML approaches have always been criticized for following a black box approach with very little clarity on the process happening inside a model, but with the advent of AutoML tools and library and with its correct amalgamation with Explainable AI (XAI), it has given a lot more clarity on the process and also much better interpretability of the models and the affect each and every variable of the dataset can have on the model. Some of the most famous and widely used AutoML libraries are AutoViML, PyCaret, Auto sklearn, Auto keras, Tree-Based Pipeline Optimization, etc.
Provides a detailed explanation of Data Science concepts, methods and algorithms, all reinforced by practical examples that are applied to genomics
Presents a roadmap of future trends suitable for innovative Data Science research and practice
Includes topics such as Blockchain technology for securing data at end user/server side
Presents real world case studies, open issues and challenges faced in Genomics, including future research directions and a separate chapter for Ethical Concerns
Скачать Data Science for Genomics

[related-news] [/related-news]
Внимание
Уважаемый посетитель, Вы зашли на сайт как незарегистрированный пользователь.
Мы рекомендуем Вам зарегистрироваться либо войти на сайт под своим именем.
Уважаемый посетитель, Вы зашли на сайт как незарегистрированный пользователь.
Мы рекомендуем Вам зарегистрироваться либо войти на сайт под своим именем.