Computing for Data Analysis: Theory and Practices
- Добавил: literator
- Дата: 5-02-2023, 18:27
- Комментариев: 0
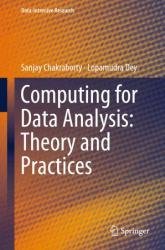
Автор: Sanjay Chakraborty, Lopamudra Dey
Издательство: Springer
Год: 2023
Страниц: 230
Язык: английский
Формат: pdf (true), epub
Размер: 25.2 MB
This book covers various cutting-edge computing technologies and their applications over data. It discusses in-depth knowledge on big data and cloud computing, quantum computing, cognitive computing, and computational biology with respect to different kinds of data analysis and applications. In this book, authors describe some interesting models in the cloud, quantum, cognitive, and computational biology domains that provide some useful impact on intelligent data (emotional, image, etc.) analysis. They also explain how these computing technologies based data analysis approaches used for various real-life applications. The book will be beneficial for readers working in this area.
Data analytics is significant since it aids in the performance optimization of enterprises. By finding more cost-effective ways to do business and retaining a lot of data, firms can help cut expenses by incorporating it into their business strategy. Analyzing data collections to identify trends and make judgments about the information they contain is known as Data Analytics (DA). Data analytics is increasingly carried out with the use of specialist hardware and software.
This book has covered various cutting-edge computing technologies and their applications over data. We have discussed in-depth knowledge of big data and cloud computing, the Internet of Things, augmented and virtual reality, quantum computing, cognitive computing, and computational biology with respect to different kinds of data analysis and their applications. In this book, we have described some interesting models in the cloud, IoT, AR/VR systems, quantum, cognitive, and computational biology domains that provide some useful impact on intelligent data (bulk time series data, emotional, image data, etc.) analysis. We have also explained how these computing technologies-based data analysis approaches are used for various real-life applications. We believe this book will be benefited the readers who are interested to work in these areas in the future.
Chapter 1 gives an overall introduction to the basics of big data analytics, cloud data analytics, quantum, and IoT-based data analytics, biological data analytics, and so on. It described the impact of data analysis on all these frameworks briefly.
Then under Part I, Chap. 2 discusses the roles and different techniques of big data analysis on a cloud platform. It first discusses the various types of big data analysis and how that data analysis can be performed in Hadoop architecture through a cloud framework. To find patterns in data and derive fresh insights, cloud analytics entails the combination of scalable cloud computing with robust analytical tools. Data analysis is being used by corporations to gain a competitive edge, enhance scientific research, and improve people’s lives in a variety of ways. Therefore, it describes the basics of the cloud, its different models, and architectures and also explains how they help to do effective data analysis.
Chapters 3 and 4 focus on the discussion of edge computing with the notions of the Internet of Things (IoT) and augmented/virtual (AR/VR) reality. Chapter 3 introduces the basic concepts of IoT along with the related technologies, protocols, and architecture. Then, it describes the impact of IoT on various industrial applications and big data analysis on cloud framework. Chapter 4 discusses the types of augmented reality with some specific system architectures. It also explains the different hardware and software components of AR/VR systems. It also presents the different real-life applications of data analysis and future research directions in this area.
Chapter 5 under Part II takes a more in-depth look at data analysis in the Biocomputing domain. In this domain, we discuss the basic concepts of computational biology and its various data types. Besides that, it describes the different data analysis processes on DNA/RNA sequences, microarray data sequences, and protein sequences.
Chapter 6 discusses the data analysis through cognitive computing. In this chapter, we describe the basics of brain–computer interfacing techniques for feature extraction and its various components. It also presents the methodology for the classification of emotional data through the analysis of EEG signals collected from the human brain. It has huge applications for those people who are getting distressed due to work pressure or other issues in their day to day life.
In Part III, Chaps. 7 and 8 deal with the concepts of quantum computing that help to perform various machine learning and image processing operations on a set of real-life data and image matrices. Chapter 7 discusses the basics of quantum machine learning concepts and how they can be utilized to solve some complex clustering and classification problems more efficiently compared to classical computing. Similarly, two important and complex image processing operations (denoising and edge detection) that can be solved more efficiently and faster way in the quantum framework are discussed in Chap. 8.
Finally, Chap. 9 under Part IV summarizes the concepts presented in this book and discusses applications and trends in data analysis. Social impacts of data analysis, such as privacy and data security issues, are discussed, in addition to challenging research issues.
Скачать Computing for Data Analysis: Theory and Practices

[related-news] [/related-news]
Внимание
Уважаемый посетитель, Вы зашли на сайт как незарегистрированный пользователь.
Мы рекомендуем Вам зарегистрироваться либо войти на сайт под своим именем.
Уважаемый посетитель, Вы зашли на сайт как незарегистрированный пользователь.
Мы рекомендуем Вам зарегистрироваться либо войти на сайт под своим именем.