Statistics at Square Two, 3rd Edition
- Добавил: literator
- Дата: 20-02-2023, 03:10
- Комментариев: 0
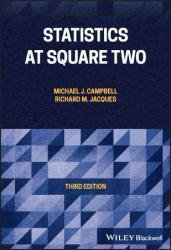
Автор: Michael J. Campbell, Richard M. Jacques
Издательство: Wiley
Год: 2023
Страниц: 208
Язык: английский
Формат: epub (true)
Размер: 10.1 MB
An easy-to-follow exploration of intermediate statistical techniques used in medical research.
In the newly revised third edition of Statistics at Square Two: Understanding Modern Statistical Applications in Medicine , a team of distinguished statisticians delivers an accessible and intuitive discussion of advanced statistical methods for readers and users of scientific medical literature. This will allow readers to engage critically with modern research as the authors explain the correct interpretation of results in the medical literature. The book includes two brand new chapters covering meta-analysis and time-series analysis as well as new references to the many checklists that have appeared in recent years to enable better reporting of contemporary research. Most examples have been updated as well, and each chapter contains practice exercises and answers. Readers will also find sample code (in R) for many of the analyses, in addition to:
A thorough introduction to models and data, including the different types of data, statistical models, and computer-intensive methods
Comprehensive explorations of multiple linear regression, including the interpretation of computer output, diagnostic statistics such as influential points, and many uses of multiple regression
Practical discussions of multiple logistic regression, survival analysis, Poisson regression and random effects models including their uses, examples in the medical literature, and strategies for interpreting computer output
Since most researchers do not want to become statisticians, detailed explanations of the methodology will be avoided. However, equations of the models are given, since they show concisely what each model is assuming. We hope the book will prove useful to students on postgraduate courses and for this reason there are a number of exercises with answers. The choice of topics reflects what we feel are commonly encountered in the medical literature, based on many years of statistical refereeing. The linking theme is regression models and we cover multiple regression, logistic regression, Cox regression, random effects (mixed models), ordinal regression, Poisson regression, time series regression and meta-analysis. The predominant philosophy is frequentist, since this reflects the literature and what is available in most packages. However, a discussion on the uses of Bayesian methods is given in an Appendix 4. The huge amount of work on causal modelling is briefly referenced, but is generally beyond the scope of this book. All the analyses described in the book have been conducted in the free software R and the code is given to make the methods accessible to reserachers without commercial statistical packages.
Causal inference is a new area of statistics that examines the relationship between a putative cause and an outcome. A useful and simple method of displaying a causal model is with a Direct Acyclic Graph (DAG).1 They can be used to explain the definitions given in the previous section. There are two key features to DAGs: (1) they show direct relationships using lines and arrows and are usually read from left to right and (2) they don’t allow feedback, that is, you can’t get back to where you started following the arrows.
Perfect for anyone hoping to better understand the statistics presented in contemporary medical research, Statistics at Square Two: Understanding Modern Statistical Applications in Medicine will also benefit postgraduate students studying statistics and medicine.
Table of Contents:
Preface
1 Models, Tests and Data
2 Multiple Linear Regression
3 Multiple Logistic Regression
4 Survival Analysis
5 Random Effects Models
6 Poisson and Ordinal Regression
7 Meta-analysis
8 Time Series Regression
Appendix 1 Exponentials and Logarithms
Appendix 2 Maximum Likelihood and Significance Tests
Appendix 3 Bootstrapping and Variance Robust Standard Errors
Appendix 4 Bayesian Methods
Appendix 5 R codes
Answers to Exercises
Glossary
Index
Скачать Statistics at Square Two, 3rd Edition

[related-news] [/related-news]
Внимание
Уважаемый посетитель, Вы зашли на сайт как незарегистрированный пользователь.
Мы рекомендуем Вам зарегистрироваться либо войти на сайт под своим именем.
Уважаемый посетитель, Вы зашли на сайт как незарегистрированный пользователь.
Мы рекомендуем Вам зарегистрироваться либо войти на сайт под своим именем.