Mathematical Modelling of Contemporary Electricity Markets
- Добавил: literator
- Дата: 26-04-2021, 18:53
- Комментариев: 0
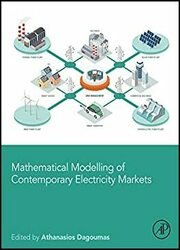
Автор: Athanasios Dagoumas
Издательство: Academic Press, Elsevier
Год: 2021
Страниц: 422
Язык: английский
Формат: pdf (true)
Размер: 13.4 MB
Mathematical Modelling of Contemporary Electricity Markets reviews major methodologies and tools to accurately analyze and forecast contemporary electricity markets in a ways that is ideal for practitioner and academic audiences. Approaches include optimization, neural networks, genetic algorithms, co-optimization, econometrics, E3 models and energy system models. The work examines how new challenges affect power market modeling, including discussions of stochastic renewables, price volatility, dynamic participation of demand, integration of storage and electric vehicles, interdependence with other commodity markets and the evolution of policy developments (market coupling processes, security of supply). Coverage addresses all major forms of electricity markets: day-ahead, forward, intraday, balancing, and capacity.
Who is the target audience.
The book captures mainly the needs of energy experts; however, it could also be useful as a textbook, especially in postgraduate studies and for doctoral students. Therefore the target audience is:
Energy experts working with electricity companies, system operators, energy exchanges, and regulators
Master/PhD students expanding their quantitative skills for competitive electricity markets
Analysts in the power sector and in academia
Electricity market analysts, electricity traders, heads of energy management and energy trading division, electricity
retailers, risk analysts in banking and in electric utilities, energy investors
Chapter 5 focuses on forecasting of week-ahead hourly electricity prices in Belgium with statistical and Machine Learning (ML) methods. Electricity price forecasting is influenced by numerous variables, such as weather conditions, electricity consumption, and seasonal factors. This chapter provides an overview of both statistical and ML approaches. It compares the forecasting performance of two popular ML methods, namely neural networks and random forest, to that of traditional, statistical ones by considering the electricity market in Belgium. The results indicate that ML methods provide better forecasts, both in terms of accuracy and bias. External variables improve the performance of both statistical and ML approaches; however, the improvements in the latter group are relatively more substantial.
Chapter 11 focuses on electricity portfolio optimization through the development of a Mixed Integer Linear Programming (MILP) model. The chapter proposes an optimization-based methodological framework for the optimal economic portfolio management of a provider. The latter has the ability to produce electricity from its natural gas power plant, buying from the day-ahead spot market or curtailing part of the demand through Demand Response (DR) programs.
The book aims to improve the understanding of the abovementioned personnel in power markets operations, to improve their modelling capabilities for electricity markets as well as the understanding of the modelling outputs, to improve their strategy in participation in electricity markets, and to improve their understanding in decision making in energy investments.
Скачать Mathematical Modelling of Contemporary Electricity Markets

[related-news] [/related-news]
Внимание
Уважаемый посетитель, Вы зашли на сайт как незарегистрированный пользователь.
Мы рекомендуем Вам зарегистрироваться либо войти на сайт под своим именем.
Уважаемый посетитель, Вы зашли на сайт как незарегистрированный пользователь.
Мы рекомендуем Вам зарегистрироваться либо войти на сайт под своим именем.