Machine Learning for Drone-enabled Iot Networks: Opportunities, Developments, and Trends
- Добавил: literator
- Дата: Сегодня, 09:24
- Комментариев: 0
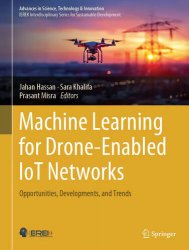
Автор: Jahan Hassan, Sara Khalifa, Prasant Misra
Издательство: Springer
Год: 2025
Страниц: 213
Язык: английский
Формат: pdf
Размер: 15.8 MB
This book aims to explore the latest developments, challenges, and opportunities in the application of machine learning techniques to enhance the performance and efficiency of IoT networks assisted by aerial unmanned vehicles (UAVs), commonly known as drones. The book aims to include cutting edge research and development on a number of areas within the topic including but not limited to: •Machine learning algorithms for drone-enabled IoT networks •Sensing and data collection with drones for IoT applications •Data analysis and processing for IoT networks assisted by drones •Energy-efficient and scalable solutions for drone-assisted IoT networks •Security and privacy issues in drone-enabled IoT networks •Emerging trends and future directions in ML for drone-assisted IoT networks.
Gives latest in ML for drone-assisted IoT networks from experts, including practical insights and solutions
Aims to explore latest developments, challenges, and opportunities in the application of machine learning techniques
Includes cutting edge research and development on a number of areas
The first chapter “Unmanned Aerial Vehicles Enabled IoT Platform for Effective Disaster Management” explores the transformative role of Unmanned Aerial Vehicles (UAVs) integrated with Internet of Things (IoT) platforms in disaster management. It emphasizes their capacity for real-time data collection and enhanced situational awareness during emergencies, illustrating how these technologies can detect anomalies and support informed decision-making. The chapter introduces advancements in UAV–IoT networks and outlines various approaches to system architecture and data collection, featuring algorithms such as YOLO and LSTM. Additionally, it addresses critical challenges, including security, privacy, and network coverage while providing guidelines for optimizing these technologies in disaster response. Looking ahead, the first chapter “Unmanned Aerial Vehicles Enabled IoT Platform for Effective Disaster Management” envisions potential advancements in AI, deep learning, and blockchain technology, highlighting their role in improving the efficiency and effectiveness of disaster management. This comprehensive overview aims to illuminate the current and future impact of UAV-enabled IoT platforms in emergency scenarios.
The second chapter “Exploring Machine and Deep Learning for Crop Detection and Yield Prediction in Drone-Enabled IoT Networks” explores the transformative advancements in agriculture driven by the integration of drones and Internet of Things (IoT) platforms. It highlights how these technologies have revolutionized traditional practices, turning labor-intensive tasks like crop counting and production estimation into efficient, data-driven processes. By leveraging Machine Learning (ML) and Deep Learning (DL) models, the chapter demonstrates effective strategies for analyzing agricultural data tailored to specific factors such as crop types and image resolutions. Through a focused study on managing apricot crops, it showcases the capabilities of YOLOv10, which achieved an impressive F1 Score of 88%, and a linear regression model for yield prediction with a low Relative Error of 2.18%. This exploration underscores the remarkable potential of ML and DL technologies to revolutionize precision agriculture and enhance productivity.
In the third chapter “Drone Swarm Coordination Using Machine Learning in IoT Networks”, authors explore the transformative potential of drone swarm coordination using machine learning in IoT networks. As Unmanned Aerial Vehicles (UAVs) revolutionize the aviation industry, the concept of UAV swarms enables multiple drones to operate autonomously, executing tasks without human intervention. This chapter underscores the critical role of communication in managing these swarms, highlighting the need for robust systems to tackle challenges such as coordination, control, and security. By leveraging distributed learning algorithms, drone swarms can enhance capabilities like target tracking, remote surveillance, and efficient resource allocation.
Скачать Machine Learning for Drone-enabled Iot Networks: Opportunities, Developments, and Trends

[related-news] [/related-news]
Внимание
Уважаемый посетитель, Вы зашли на сайт как незарегистрированный пользователь.
Мы рекомендуем Вам зарегистрироваться либо войти на сайт под своим именем.
Уважаемый посетитель, Вы зашли на сайт как незарегистрированный пользователь.
Мы рекомендуем Вам зарегистрироваться либо войти на сайт под своим именем.